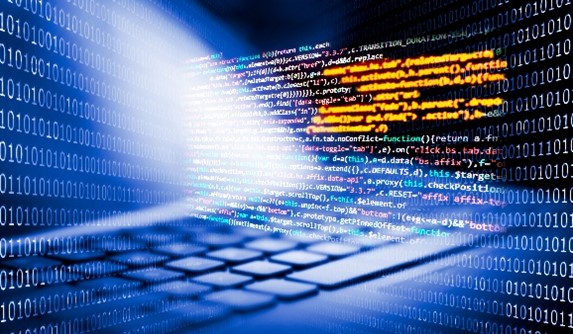
Controversy swirls around the use of the term AI which, like so many other popular terms, does not have a universally accepted definition. A Google search for “healthcare AI” yields 163 million results. Even users of AI have different views on what the “A” in AI means – artificial intelligence or augmented intelligence? Both can be accurate and useful depending on context and audience. The opportunities they present are endless. AI and AI are fueling innovation and instantiation of evidence-based best practices associated with digital health systems.
According to Artificial Solutions, “It was in the mid-1950s that [John] McCarthy coined the term ‘Artificial Intelligence’ which he would define as ‘the science and engineering of making intelligent machines’.” Science Focus reports, “Instead of an artificial intelligence, the idea of augmenting our own intelligence with technology was first proposed in 1960 by an American psychologist and computer scientist called Joseph Carl Robnett Licklider, in an important article titled ‘Man-computer symbiosis’.” Regardless of one’s preference, the concepts associated with both versions of AI continue to inspire developments and usage that will further the efforts of those involved in Digital Health.
Two primary areas where AI (in either form) can be deployed are clinical and administrative. There are many common elements in the governance process but there are some differences that must be addressed to ensure efficacy and efficiency for each.
Development and deployment of AI that will be used to tech-enable clinical care should follow already well-defined governance processes for human experimentation and clinical trials. At the top of the governance process should be the equivalent of an IRB (institutional review board). That recommendation may seem onerous, but an AI algorithm is software that in the broadest sense may be viewed as a medical device. Hopefully, during the design process for an AI algorithm, a review by physicians, researchers, ethicists, security and privacy teams, and patients and families will take place. That should help expedite the final approval process.
For both clinical and administrative AI, you should ensure that your design, development, testing, and validation efforts address DEI (diversity, equity, and inclusion) very deliberately. The absence of champions from those areas will dramatically increase the chance of bias – the consequences of which could be dramatic. In curriculum development and training for AI-enabled applications, particularly if there is competency testing required in order to get your credentials, there must be sensitivity to DEI issues.
Of the topics that deserve attention, too often, security, privacy, and ethics are often overlooked until deployment. That’s too late. Address these topics as part of the design. They are foundational elements for the build and should be treated as essential requirements for operations. To avoid costly and sometimes insurmountable challenges, be deliberate about security, privacy, and ethics during all phases of development – from design through deployment.
Ravi Parikh, MD, MPP and his associates published a very useful article in JAMA. Here are key takeaways described on HealthDataManagement.com based on their work and an article in Science that was published in 2019 which quoted tweets by Dr. Parikh.
- “Just like drugs and devices, show evidence of clinical (not just statistical) benefit.”
- “…benchmark algorithms against standards of care…”
- “Make sure these tools are better than clinicians’ intuition (which is pretty good).”
- Validate the result at many facilities, not just a “single institution in specific populations.”
- “Specify the interventions. Better predictive tools only improve care if we know how to act on [them]. In many prospective studies, the intervention that led to clinical benefit was more important than the algorithm prediction.”
- “…do post-marketing studies.”
Dr. Parikh points out that as the algorithm rendered by machine learning evolves, it may impact the algorithm; hence, it is important to revisit and test the validity of the underlying logic.
For financial and administrative areas, the governance mechanism can and should be minimized. For the design, development, testing, and validation processes, you will still want to call together multi-entity, multidisciplinary teams with representatives from affected areas – directly or indirectly – to ensure that you’re representing all constituencies.
One cannot overstate the value of the synergies that come from the conversations that accompany the governance processes where a diverse group of stakeholders collaborates to identify, explore, and provide practical and pragmatic AI solutions for innumerable healthcare challenges. AI and AI should not be seen as ways to replace humans but as technologies that allow us to instantiate learnings from the human experience. AI applied responsibly and compassionately allows people to share evidence-based practices and focus on the more human-oriented tasks that cannot be automated. Importantly, AI helps us recover time to spend on our community, our providers, our patients, their families, and ourselves.